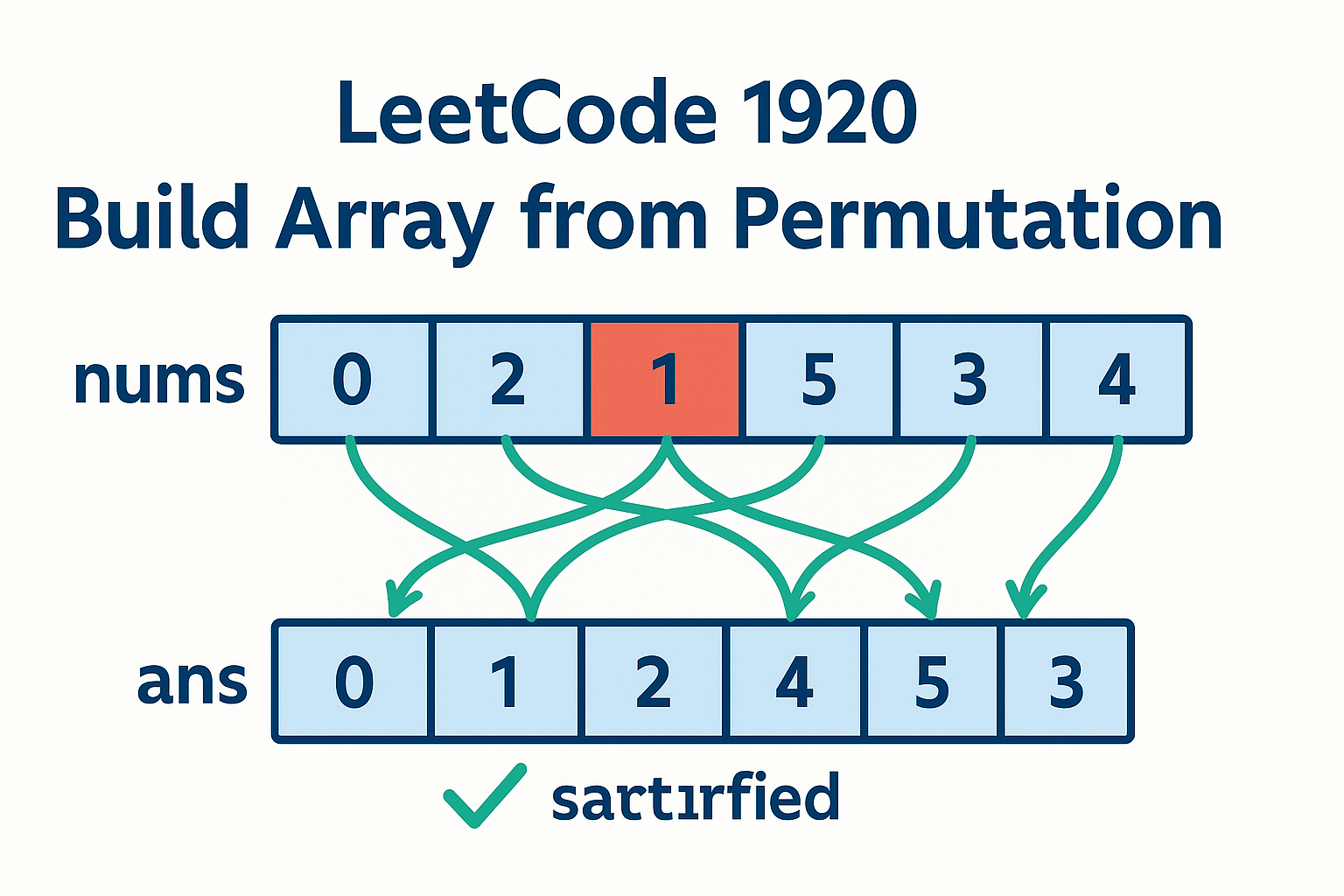
1920: Build Array from Permutation
Problem Statement
Given a zero-based permutation array nums
, return an array ans
where ans[i] = nums[nums[i]]
for each index i
. Solve it with O(1) extra space for bonus points.
Example 1
Input: nums = [0,2,1,5,3,4]
Output: [0,1,2,4,5,3]
Explanation: Each element is nums[nums[i]]
Example 2
Input: nums = [5,0,1,2,3,4]
Output: [4,5,0,1,2,3]
Explanation: Transformation done in-place
Approach 1: Straightforward Solution (O(n) Space)
Create a new array and fill it with transformed values.
Algorithm Steps
- Initialize empty result array
- Iterate through each index
- Store nums[nums[i]] in result
Complexity Analysis
Time Complexity | Space Complexity |
---|---|
O(n) | O(n) |
class Solution {
public:
vector<int> buildArray(vector<int>& nums) {
vector<int> ans(nums.size());
for(int i = 0; i < nums.size(); ++i) {
ans[i] = nums[nums[i]];
}
return ans;
}
};
class Solution {
public int[] buildArray(int[] nums) {
int[] ans = new int[nums.length];
for(int i = 0; i < nums.length; i++) {
ans[i] = nums[nums[i]];
}
return ans;
}
}
class Solution:
def buildArray(self, nums: List[int]) -> List[int]:
return [nums[num] for num in nums]
Approach 2: In-place O(1) Space Solution
Encode two values in each position using mathematical manipulation.
Algorithm Steps
- Use formula: nums[i] += n * (nums[nums[i]] % n)
- Divide each element by n to get final values
- Modulo operation preserves original value
Complexity Analysis
Time Complexity | Space Complexity |
---|---|
O(n) | O(1) |
class Solution {
public:
vector<int> buildArray(vector<int>& nums) {
int n = nums.size();
for(int i = 0; i < n; ++i) {
nums[i] += n * (nums[nums[i]] % n);
}
for(int i = 0; i < n; ++i) {
nums[i] /= n;
}
return nums;
}
};
class Solution {
public int[] buildArray(int[] nums) {
int n = nums.length;
for(int i = 0; i < n; i++) {
nums[i] += n * (nums[nums[i]] % n);
}
for(int i = 0; i < n; i++) {
nums[i] /= n;
}
return nums;
}
}
class Solution:
def buildArray(self, nums: List[int]) -> List[int]:
n = len(nums)
for i in range(n):
nums[i] += n * (nums[nums[i]] % n)
for i in range(n):
nums[i] //= n
return nums
Approach Comparison
Approach | Time | Space | Use Case |
---|---|---|---|
Basic Solution | O(n) | O(n) | Readability, Small input |
In-place Solution | O(n) | O(1) | Large datasets, Memory constraints |
Edge Cases and Testing
1. Minimum Input (n=1)
Input: [0] → Output: [0]
2. Maximum Permutation
Input: [4,3,2,1,0] → Output: [0,1,2,3,4]
3. Cyclic Dependencies
Input: [2,0,1] → Output: [1,2,0]
Frequently Asked Questions
1. Why does the in-place method work with modulo?
Using nums[nums[i]] % n preserves the original value before any modifications during the first pass, ensuring correct calculations.
2. How does encoding two values work?
We store new_value * n + original_value in each position. Division by n extracts new value, modulo gets original (but not needed in final result).
3. What if nums contains large numbers?
The problem constraints guarantee 0 ≤ nums[i] < n, making the encoding safe within standard integer ranges.
4. Why multiply by n instead of other numbers?
Since all values are < n, multiplying by n creates a unique base-n encoding that prevents information overlap.
5. Can we solve this with bit manipulation?
Yes, but modulo/division approach is more readable. Bitwise methods would need sufficient bit space (unlikely in practice).
6. How to handle overflow?
Constraints ensure n ≤ 1000, so 1000*1000 = 1,000,000 fits in 32-bit integers (max 2,147,483,647).
7. Does order of processing matter?
Yes! Must complete first full pass before starting division pass to prevent data corruption.
8. What if nums is read-only?
Use basic O(n) space approach. The in-place solution requires write access.
9. How to verify correctness?
Check ans[i] = nums[nums[i]] for all i. Test with cyclic permutations and edge cases.
10. Why is this problem important?
Teaches advanced array manipulation techniques crucial for memory-constrained environments and low-level programming.